OnCorps has trained, tested and deployed investment operations algorithms for over five years. This gives us a unique vantage point on the differences between humans, software, and AI. While the best solutions require some combination of the three, the mistakes we see come from over- or under-focusing on the capabilities of any one in isolation. Here is our view on what has worked best in each of five core functions and decisions in investment operations.
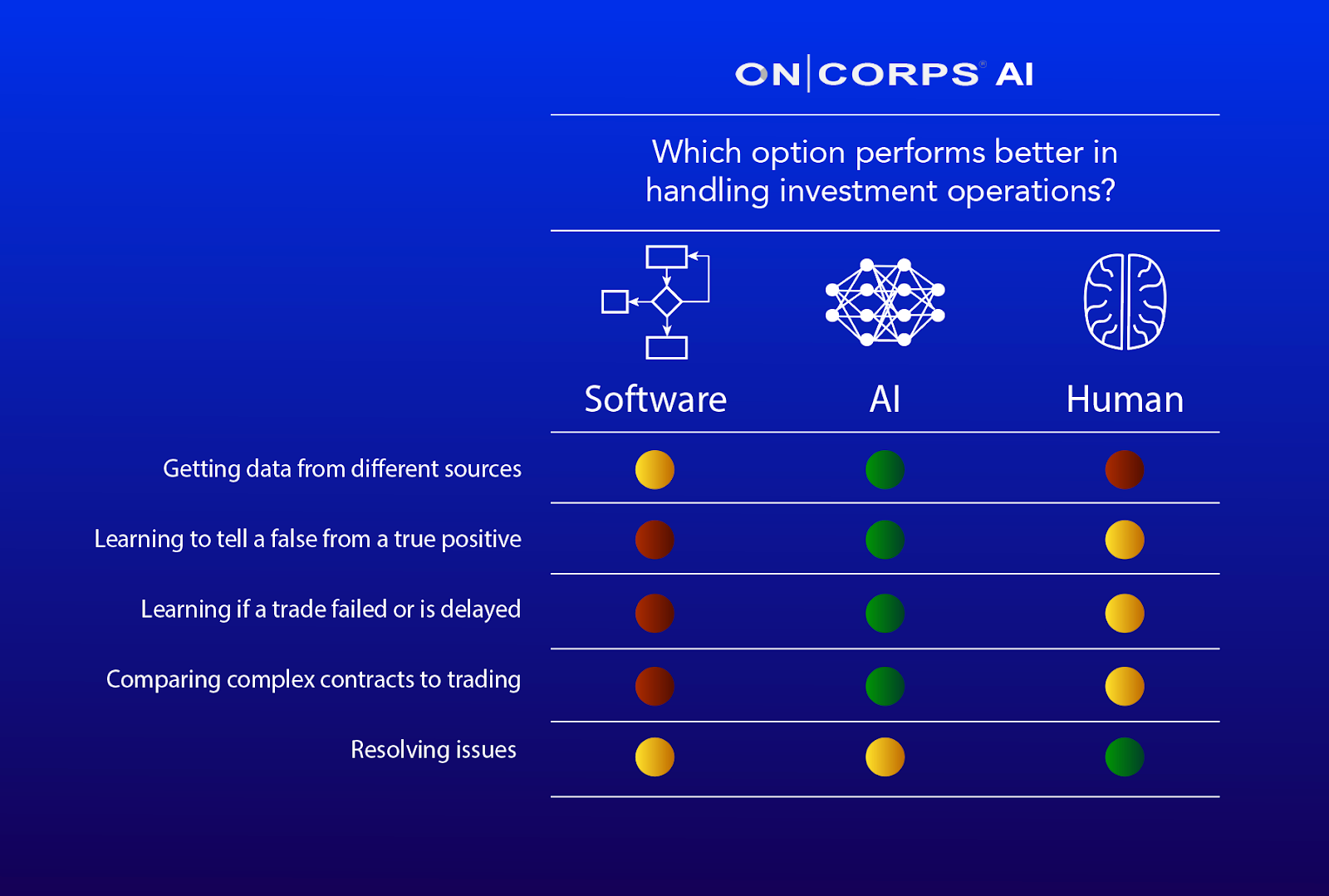
Our Assessment of Performance Across Five Tasks and Decisions
We assessed how software, humans, and AI handle five critical tasks and decisions.
- Getting data from different sources. Our systems in investment operations are only as effective as the data they compare. Investment reconciliations compare data from many different systems and formats, some changing regularly. Many leaders tell us this consumes over half their team’s time. While there have been significant advances in data management, most software-based solutions have failed to tell whether two data points represent the exact same state of a transaction. They are also challenged with understanding slight differences in data descriptions. By contrast, AI solutions such as Databricks have allowed us to map and trace patterns in lineage, sources, and descriptions. These features were originally developed to manage the governance of distributed data and algorithms. However, we have found them quite effective at mapping data from two different sources and detecting differences.
- Learning to tell a false positive from a true positive. Reconciliation systems and methods produce a significant volume of exceptions. Most of these exceptions are false positives. For example, an investment ops analyst may spend time on a potential trade fail, only to discover it was delayed. This is wasted time if they could tell the trade would be settled a day later. Traditional software can help with workflows and data management, but have failed to reduce exceptions, redundancies, and labor. AI can help reduce false positives in several ways. First, we employ anomaly detection methods to isolate anomalies across multiple variables. This is better than software which tends only to focus on value differences. Second, we use incident detection algorithms to search for the data conditions that produced past errors. Finally, we train algorithms to learn root causes so that they can improve their ability to rule out false positives sooner while not missing true errors. Combined, these algorithms have enabled a 90+ percent reduction in exceptions. For example, a recent red team test revealed that 96 percent of the exceptions flagged were deemed by a bank’s team as worth seeing.
- Learning if a trade has failed or is delayed. The combination of rising interest rates and T+1 settlement requirements puts pressure on investment operations to resolve open trades faster. Our early work in this area indicates we can break down trades by asset class and broker/dealer. Once this segmentation is done, we train algorithms to predict the settlement times of specific trades possessing different characteristics and contexts. By training algorithms to predict settlement time, much like a navigation app, we can also learn the causes of delays. We are quite encouraged by this approach and look forward to sharing more as we progress.
- Comparing complex contracts to trading. Investment operations teams spend each day reading complex legal confirmations and checking electronic records to ensure the contract and execution are aligned. Staff must learn the nuances of many different derivative, credit, and loan instruments. They learn to read both the economic and legal terms of these products. They also learn the differences between counterparty contract formats. All this knowledge is held by a few key resources in operations. We have trained AI to distinguish the product and counterparty then sort them into the right comparison buckets. Once that is done, different NLP-based algorithms extract the economic terms for comparison to electronic trading records. Legal terms are compared to variances from past contracts and highlighted as red, yellow, or green depending on the degree of change in language from the past. The system even manages the workflow and notification to various constituents such as the counterparty, legal, and investment teams. The results of our AI based system have been impressive. On average, a complex OTC confirmation takes an analyst 40 minutes to review and reconcile. Our AI system typically accomplishes the task in 1-2 minutes.
- Resolving issues. Confirming the resolution of issues is a task that requires a combination of communication and detail-orientation. To date, we think humans are still the best at performing these tasks. That said, we are developing agents who may someday be capable of autonomous exception resolution. This is more likely for exceptions with plenty of outcome data and few edge cases.
Hybrid Solutions
The reality is that all solutions will require some hybrid combination of AI, software, and humans. The challenge is balancing each of these to avoid their respective weaknesses. Software is rules-based and rigid. It doesn’t learn and can’t handle minor changes in formats. Supplementing this code with algorithms has been critical to our success in productionizing over 20 algorithms. Humans, on the other hand, aren’t consistent and exhibit behavioral vulnerabilities. For example, we detected and studied “eye glaze syndrome” with a lab at Yale University. This work showed that people begin missing true positives when exposed to too many false positives. And AI is still in its very early stages of development. As more communities share their breakthroughs, and more algorithms are productionized, the investment operations landscape will change quite dramatically.