An Environment Ripe for Errors
As a leading artificial intelligence partner to the world's largest asset managers, we see significant data on NAV errors. While error rates appear low, they can be costly for fund administrators and asset managers. With AI, we believe errors can be better predicted and prevented. For example, you can see in the chart below that we have systematically labeled NAV errors over a 15 year period. Though these are just three of multiple labels we manage, we have built a solid foundation for training algorithms to alert both asset managers and service providers of the probabilities of errors occurring.

What's driving these errors? Asset manager and service provider cost pressures have left them with outdated systems. These systems require manual workarounds to handle changes in regulatory reporting and products. Manual workarounds present fertile ground for mistakes. Cost cutting has led to a workforce with high turnover and variable levels of expertise.
Here is a scenario for a NAV error. It is late afternoon on a busy trading day. An accounting analyst is under pressure to meet a NAV deadline. They see an error message. The aging system lacks a feature to automatically allocate expenses when fund classes change. The analyst has never executed a manual allocation and makes the entry in error.
Predictable Events and Conditions
Though rare, there appear to be predictable conditions leading to most NAV errors. For example, as indicated in the illustration below, we believe many errors are caused by manual entries that occur infrequently. The chart illustrates the frequency of types of entries in blue and the sigmoidal probability of error in red. As you can see, the gap widens as more esoteric manual entries are required. This has been our observation in building oversight algorithms.
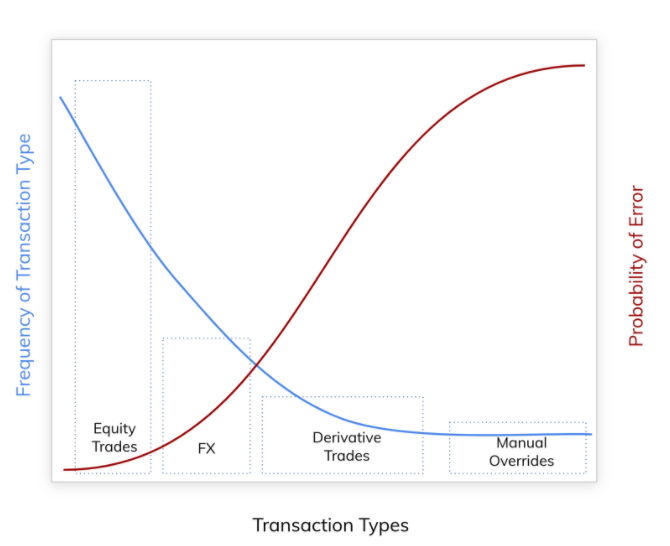
The central goal is to identify the conditions that significantly increase the probability of an error occurring before they are finalized. This requires a bottoms-up scanning and checking of all sub-ledger posting, along with advanced anomaly detection algorithms. These foundational checks continue to train our algorithm. We then intend to deliver later this year a predictive alert system.