Why Reconciliation Software Didn’t Reduce Labor
Several years ago, investment operations leaders believed better reconciliation software was key to labor productivity. Now, operations leaders we speak to are frustrated with the results. This is because software-based reconciliations still produce a high level of exceptions. Instead of manually reconciling data, the labor shifted to resolving the exceptions. While software can make it easier to track the progress of exceptions, it hasn't been effective at reducing them.
It’s a Math Problem, Not a Software Problem
We have had great success with AI techniques geared toward reducing the overall number of exceptions - sometimes by 90 percent or more. We did this by approaching the problem mathematically. In machine learning and statistics, there are two types of errors: Type 1 and Type 2. For investment operations leaders, this is quite simple. Type 1 is an exception that looked like an error, but turned out to be nothing. This is also known as a false positive and accounts for most of the labor in investment operations. Every day, middle office workers pursue explanations to suspicious trades that turn out to be simple delays. Type 2 errors are seen as non-issues and later discovered as errors. The challenge for investment operations managers is to reduce both errors.
As illustrated below, we can see that applying simple threshold breaks against all data can lead to very high false positive rates. This is a well-known statistical principle known as Bayes Theorem. As you can see with the graph to the left, if you scan all data as indicated in the blue curve, and the actual error rate is low as indicated in the red curve, the Type 1 error rate will be quite high. Airport TSA screening is an example of this problem. They screen all passengers, even though the incident rate of terrorism is low. In this case, the false positive rate is approaching 99 percent. Even worse, when red team testing is conducted (planting plastic guns), the Type 2 error rate is over 90 percent.
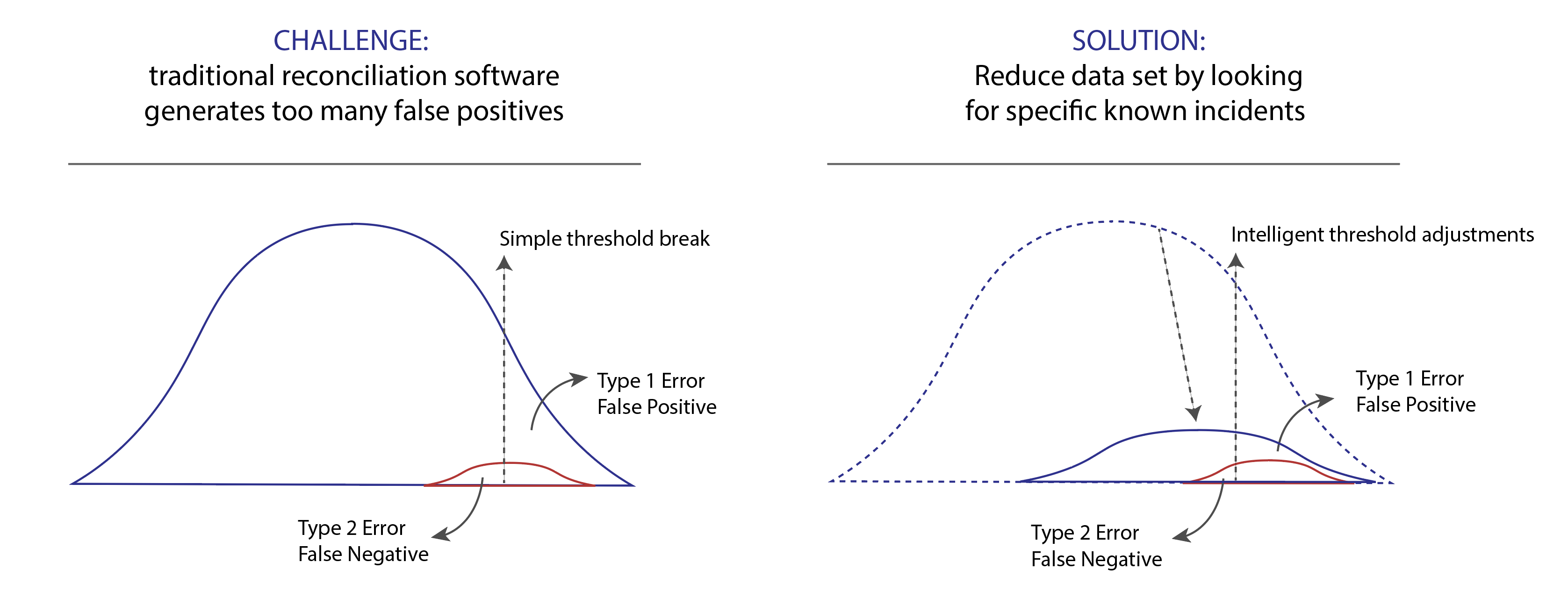
It’s Easier to Fish When You Drain the Pond
Our solution is conceptually quite simple: what if you could reduce the dataset so that the thing you are looking for is more prevalent? We have created over 20 incident detection checks, which are configured to look for the factors that caused errors in the past. For example, we run a daily incident detection check for mistakes made in accrued interest for bond purchases. The detection algorithm isolates all bond purchases first, then counts the number of days the bond is in its coupon period. These two functions eliminate many transactions and essentially operate like an intelligent filter.
Our goal is to use these checks to provide more meaningful data to learning-based algorithms. For example, we believe these may be the sensors for a more autonomous agent that can auto-resolve exceptions.
Diligent Testing With Dramatic Results
One thing we think is missing in AI is rigorous outcome testing. To address this, we solicit human-in-the-loop input from our customers on the exceptions our algorithms produce. With this feedback we can adjust our settings. We have also backtested our algorithms against historical data and asked our customers to plant known errors. We then measure two factors: the number of exceptions we produce and the percent of those exceptions that are “worth seeing.” So far, we have been able to reduce the number of exceptions produced by over 90 percent. Similarly, in a recent red team test with a major custodian bank, 94 percent of the exceptions generated were deemed by them as legitimate issues.